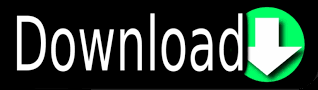

The OLS regression line 12.70286 + 0.21 X and the WLS regression line 12.85626 + 0.201223 X are not very different, as can also be seen in Figure 3.įigure 3 – Comparison of OLS and WLS regression lines Figure 2 shows the WLS (weighted least squares) regression output.įigure 2 – Weighted least squares regression The right side of the figure shows the usual OLS regression, where the weights in column C are not taken into account. Note too that if the values of the above formulas don’t change if all the weights are multiplied by a non-zero constant.Įxample 1: Conduct weighted regression for that data in columns A, B and C of Figure 1.įigure 1 – Weighted regression data + OLS regression Note thatĪs for ordinary multiple regression, we make the following definitionsĪn estimate of the covariance matrix of the coefficients is given by
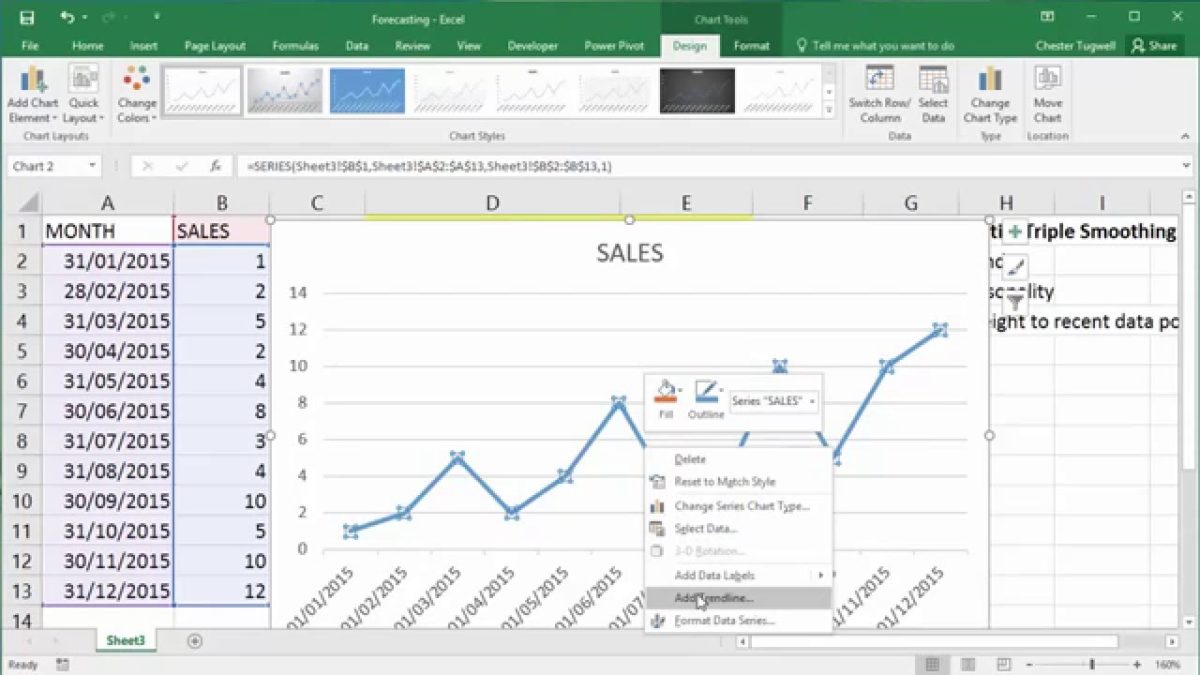
Where 1 is the n × 1 column vector consisting of all ones. We will use definitions of SS Reg and SS T that are modified versions of the OLS values, namely Also, df Reg = k and df T = n – 1, as for OLS. The n × 1 matrix of predicted y values Y-hat = and the residuals matrix E = can be expressed asĪn estimate of the variance of the residuals is given byĪs for OLS. Where W is the n × n diagonal matrix whose diagonal consists of the weights w 1, …, w n. Using the same approach as that is employed in OLS, we find that the k+1 × 1 coefficient matrix can be expressed as In weighted least squares, for a given set of weights w 1, …, w n, we seek coefficients b 0, …, b k so as to minimize Given a set of n points ( x 11, …, x 1 k, y 1), …, ( x n1, …, x nk, y n), in ordinary least squares ( OLS) the objective is to find coefficients b 0, …, b k so as to minimize
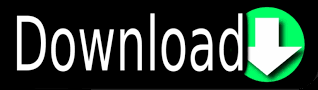